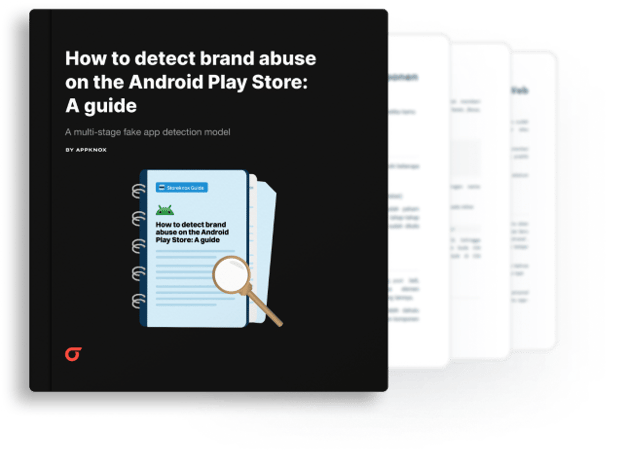
Guides
How to detect brand abuse on the Android Play Store: A guide
Learn how to detect brand abuse on the Android Play Store using a multi-stage fake app detection model. Spot impersonators, protect your brand, and stay ahead of threats.
Introduction
As mobile apps have become ubiquitous and indispensable parts of our daily lives (both professional and personal), it has created a situation that is ripe for malicious actors to exploit users - Popular apps that are in high demand are often targets of impersonation or cloning. Malicious actors create listings on app stores to distribute ‘fake apps’ that mimic legitimate apps.
Today, fake apps are one of the biggest cybersecurity threats. Such apps can cause significant harm to users, including theft of personal data, financial loss, and device compromise.
How widespread is the issue? In 2024 alone, Google blocked 2.36 million apps that were suspected to be fake from the Play Store.
But app development and security teams face two key challenges:
- Malicious actors can create fake app listings that are virtually indistinguishable from the real app - How can you detect fake apps on the Android Play Store?
- The sheer volume of fake apps on the Play Store makes manual vetting practically impossible - How can you automate the detection of fake apps?
The Appknox Solution: A multi-stage fake app detection model
At Appknox, we developed a model to help app developers and security teams accurately identify fake apps on the Android Play Store.
Our security experts identified certain recurring patterns and features that are typically found in fake apps. We analyzed these patterns to build a probability model that can determine whether an Android application is fake or authentic.
Here’s a breakdown of our fake app detection model:
- First, we provided the recurring features as parameters (malware signatures, certificate properties, requesting for dangerous permissions, etc.) to a model that screens applications and scores them on a scale that indicates the probability of an app being fake/malicious.
- Next, we score an authentic app on these parameters, and compare its score against that of all publicly available applications. This helps identify any apps that are trying to disguise themselves as the original.
- The model concurrently checks Play Store data. For instance, fake/malicious apps tend to grow in popularity faster than legitimate apps. If leading sessions numbers and sentiment analysis scores for an app seem artificially inflated, chances are it is a fake app.
- Additionally, the model checks for other markers for fake apps:
- the certificate attributes of an application cannot be the same as that of the CA issuing the certificates
- Start date and end date of a certificate of two applications cannot be the same
So in a nutshell, the Appknox fake app detection model
- analyzes immutable parameters in the application code, and
- analyzes Play Store data for indicators of suspicious activities
The model assigns a weight to each parameter to create a proprietary formula.
This formula produces a score that indicates the probability of an app being malicious or fake.
Detecting fake apps on the Play Store: Defining the Parameters
The model uses over 20 parameters, each carrying a specific weight.
-Parameters that are strong indicators of fake apps, such as malware signatures or mismatched certificate attributes, have higher weights.
-Parameters that are less conclusive, such as blocked regions or application name similarities, are assigned lower weights.
Here’s a complete list of the parameters used by the detection model:
- Application Name: Attackers use typo squatting, incorrect spellings, special characters, and different fonts to trick users.
- Application Logo: Checking for deviations from the original app's logo
- Developer Information: Ensuring the developer matches the authentic app's developer.
- Application Description: Looking for duplicate descriptions across apps with different developers.
- Application Size: Checking for significant deviations in size, which could indicate added malicious code or missing features.
- Update Frequency: Determining if the app has regular updates like the original.
- Number of Downloads: Comparing the number of downloads to the original application.
- Version History: Analyzing the version history for inconsistencies and deviations from the original app's versioning scheme.
- Screenshots: Comparing screenshots to detect copied or altered images.
- Reviews (Sentiment Analysis): Analyzing reviews to identify negative feedback indicative of fraudulent behavior.
- Permissions: Examining requested permissions, especially dangerous ones, and comparing them to the original app's permissions.
- Certificate Attributes: Verifying the authenticity and uniqueness of the application's certificate attributes.
- Development Platform: Determining the platform used to develop the app and comparing it to the original.
- Intents: Analyzing the intents (especially implicit intents) for potentially malicious requests to the device or other applications.
- Hardcoded URLs: Detecting connections to servers controlled by malicious actors.
- SBOM (Software Bill of Materials): Comparing the open-source and third-party components to identify missing functionalities.
- Suspicious Permission Patterns: Looking for illogical groupings of permissions, unrelated permissions, or unusual timing for permission requests.
- Deprecated Components: Checking for the usage of deprecated and vulnerable components.
- Sensitive APIs: Detecting the usage of sensitive APIs (e.g. exec(), runtime) in unexpected contexts.
Detection Mechanism
After defining all of the parameters in the proposed model, we create a detection mechanism:
- The model scans all the applications on the Play Store to identify potential fake and malicious applications trying to emulate a given authentic application.
- The model creates a proprietary formula for each stage of the detection process.
- Formulas from all stages are combined to create a complete function. This gives us a probabilistic score of a given application being a fake app that is trying to emulate the original application.
How the fake app detection model works
The Appknox model focuses on comparing a "testing app" to an "authentic app" to identify deviations. The model is broken down into four distinct stages:
Stage 1: Play Store Data Analysis (Filtering and Processing)
In this stage, the model uses publicly available information to quickly filter the vast number of apps down to a smaller, more manageable set of potentially fake apps.
Only those parameters that can be easily verified using publicly available data are added in this stage. Therefore, the parameters used in this stage will have relatively lower weightage in the final formula. These parameters include:
- Name of the application
- Regions where the application is blocked
- App description
- Developers of the application
Next, the model analyzes the Play Store data related to the shortlisted apps. The model compares the following parameters to identify deviations in each testing app from the authentic app’s data:
- App Size
- Frequency of Updates
- Number of Downloads
- Version History
- App Logo
- Comparison of screenshots uploaded to the listing in the Play Store
The model also performs a sentiment analysis on the user reviews of the shortlisted apps. User reviews are a good indicator of fake apps - because fraudulent or malicious apps typically have a high number of negative reviews.
After this process of elimination, we will be left with a handful of apps with a high probability of being fake or malicious.
Stage 2: Definitive Indicators of Malice (Code Analysis - Permissions, Certificates, Malware):
Next, the model shifts its focus to detecting more definitive indicators of an app being fake or malicious. The parameters analyzed in this stage are difficult to mimic. Therefore, being flagged at this stage is a high probability indicator of an app being fraudulent.
The model performs in-depth analysis of the application's code and associated attributes to identify definitive indicators of fake or malicious apps:
- General Permissions
- Special Permissions
- Certificate Attributes
- Malware and Ransomware Analysis
- Shared User ID
- Intents
- Hidden files
- Static Blocks
- Grouping of suspicious permission patterns
Stage 3: Behavioral Analysis (Network Connections, Data Access):
The earlier stages have helped us identify apps with a high probability of being fake. Now we need to confirm which apps are fake.
During this stage, the model analyzes the APK and checks for fraudulent behaviour by monitoring: which servers the app is connecting to, and what data the app is requesting from the host Android device and from other applications.
The objective of this stage is to validate the suspicions flagged in the previous stages.The parameters analyzed in this stage include:
- Content Provider Permissions
- Hardcoded URLs
- System Calls
- SBOM Analysis
- Platform on which the application was developed
- Hash comparison
- Deprecated Components with known vulnerabilities
- Nested APKs
- Sensitive APIs
Stage 4: Manual verification
In the final stage, we manually verify the apps flagged in the previous stages. The purpose is to reduce false positives and to improve the accuracy of the detection model.
During this stage, all the data from the previous stages is verified:
- How an app scored on each stage of the detection model
- Which probable and definitive indicators flagged an app
- Which apps were confirmed as fake or malicious by
The insights from this stage are used to improve the detection mechanism and filtration process in the previous stages, making the model iteratively more robust over time.
Key Parameters and Analysis Techniques
The model leverages a wide array of parameters, with specific analytical techniques:
- Application Name and Logo: Uses typo squatting detection, special character identification, different font detection and image comparison to detect similarities and deviations from the authentic app.
- Developer Information: Verifies developer consistency between the authentic and testing app. A mismatch indicates a high probability of a fake app.
- Permissions Analysis: Compares the permissions requested by the testing app with those of the authentic app, paying close attention to dangerous or special permissions that may not be related to the functionality of the app.
- Certificate Attributes: Analyzes the X.509 certificate's Subject field (CommonName, OrganizationalUnit, Organization, etc.) for discrepancies. Certificate attributes of an app cannot be copied, therefore a mismatch indicates a fake app.
- Malware Analysis: Scans the application's code for known malware signatures. Any test application containing a known malware signature will automatically be categorized as a malicious application
- Hardcoded URLs: Extracts and compares hardcoded URLs within the app to identify connections to attacker-controlled servers. A deviation in URLs is an indicator that the application is a fake trying to steal credentials and other sensitive information and storing it in an attacker controlled domain.
- Intents: Analyzes implicit intents for potentially malicious inter-application communication. Most fake apps are designed to communicate with and steal data from other apps installed on a user’s smartphone. Implicit intents are therefore a strong indicator of a fake app.
- SBOM (Software Bill of Materials) Analysis: Compares the open-source and third-party components used in the testing app with those in the authentic app. A significant deviation suggests a fake app.
- Static Blocks: Malicious code in a static block runs as soon as the class is loaded, potentially before any other code in the application.
Conclusion
Counterfeit apps that mimic legitimate apps can inflict severe damage to your brand’s reputation. Our multi-stage fake app detection model combines publicly available information with in-depth code analysis, making it a comprehensive solution to detect counterfeit apps and safeguard against brand abuse.
The use of weighted parameters and a staged filtering process makes it a highly accurate and scalable detection model. Finally, the manual verification stage acts as a crucial safeguard against false positives and ensures the detection model continuously improves over time.
Enterprises can employ this model to effectively identify and filter out fake and malicious applications, and protect their users from potential harm.